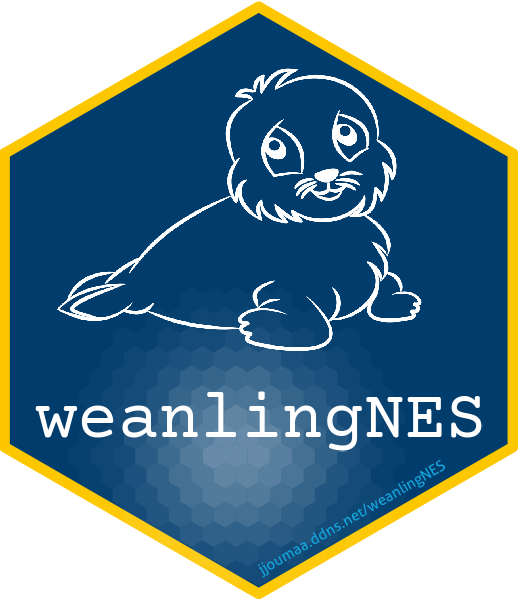
Data Comparison - NES vs SES
Joffrey JOUMAA
October 21, 2022
data_comparison_nes_ses.Rmd
Import Data
# load wealingNES package
library(weanlingNES)
# load dataset
data("data_nes")
data("data_ses")
# merge into one dataset
data_comp <- rbind(
rbindlist(data_nes$year_2018),
rbindlist(data_ses$year_2014),
use.names = T,
fill = T
)
# remove outlier (i.e. dive duration > 5000 s)
data_comp <- data_comp[dduration < 5000,]
data_comp[, diff_days := c(0,diff(day_departure)), by=.id]
# keep the first trip
data_comp_split = split(data_comp, by = c(".id"))
data_comp_split_list = lapply(data_comp_split, function(x){
# calculate diff_days
x[, diff_days := c(0,diff(day_departure)), by=.id]
# find the rows after 100 day_departure where diff_days > 5
date_cut = x[day_departure > 100 & diff_days > 1, min(date)]
# return
return(x[date < date_cut, ])
})
data_comp = rbindlist(data_comp_split_list)
# useful function to plot a dive profile based on animal ID and divenumber
plot_dive <- function(dataset){
# retrieve ind
ind = dataset[, unique(.id)]
# retrieve the time of the beginning of the dive
beg_date = dataset[, first(date)]
# species
species = dataset[, unique(sp)]
# if its a ses
if (species == "ses"){
# retrieve file
list_files = list.files("./inst/extdata/",
pattern = "*iknos_raw_data.csv",
full.names = T)
# find the one associated with ind
file_ind = grep(ind, list_files, value=TRUE)
# import file
data = fread(file_ind,
drop = "depth",
col.names = c("depth","date"))
# convert date
data[, `:=` (date = matlab_to_posix(date, timez = "GMT"))]
} else {
# retrieve file
list_files = list.files("./../Weanling Data/ucsc/Weanling Data GOOD/",
pattern = "*.csv",
recursive = T,
full.names = T)
# find the one associated with ind
files_ind = grep(paste0(substr(ind,5,11), "_nese0000annu_"),
list_files,
value=TRUE)
# keep the shorter one
file_ind = files_ind[which.min(unlist(lapply(files_ind,nchar)))]
# import file
data = fread(file_ind,
drop = c("External Temperature", "Light Level"),
col.names = c("date","time","depth"))
# convert date
data[, `:=` (date = as.POSIXct(paste(date, time),
format = "%d-%b-%Y %T",
tz = "GMT"),
time = NULL)]
}
# plot
p1 <- ggplot(data = data[date %between% c(beg_date, beg_date + (60 * 30)),],
aes(x = date, y = depth)) +
scale_y_reverse() +
geom_line() +
labs(y = "Depth (m)",
x = "Time",
title = paste0(ind, " (", species, ") - ", beg_date))
# return
return(p1)
}
Plots
Proportion of Dives
# calculate the proportion of dive for each divetype, phase, sp, .id
prop_dive_id_phase_divetype_sp <- data_comp[,table(divetype,sp,phase,.id)] %>%
# the calculate the proportion of dive in each divetype, per sp and phases
prop.table(., c(".id")) %>%
# convert into a data.table
as.data.table(.)
# merge this table to add the number of dives, per divetype, phase, sp, .id
dataPlot <- merge(prop_dive_id_phase_divetype_sp,
data_comp[,.(nb_dives = uniqueN(divenumber)),
by = .(divetype, sp , phase, .id)],
by = c("divetype","sp","phase",".id")) %>%
# mean + standard deviation
.[, .(N = wtd.mean(N, nb_dives),
N_sd = sqrt(wtd.var(N,nb_dives))), by = .(divetype,sp,phase)]
Result 1: Larger proportion of drift dives during the days compared to the night in nes, unlike ses Result 2: More transit in nes vs. more foraging in ses
# plot
ggplot(dataPlot, aes(x = divetype, y = N, fill = sp)) +
geom_bar(stat = "identity", position = "dodge") +
geom_errorbar(aes(ymin=N-N_sd, ymax=N+N_sd), width=.2,
position=position_dodge(.9)) +
scale_y_continuous(labels = label_percent()) +
facet_grid(.~phase) +
theme_jjo() +
scale_fill_manual(values = c("#e08214", "#8073ac")) +
labs(x = "Dive Types", y = "Average Proportion of Dives", fill = "Species") +
theme(legend.position = "top",
axis.line.x = element_line(arrow = NULL))
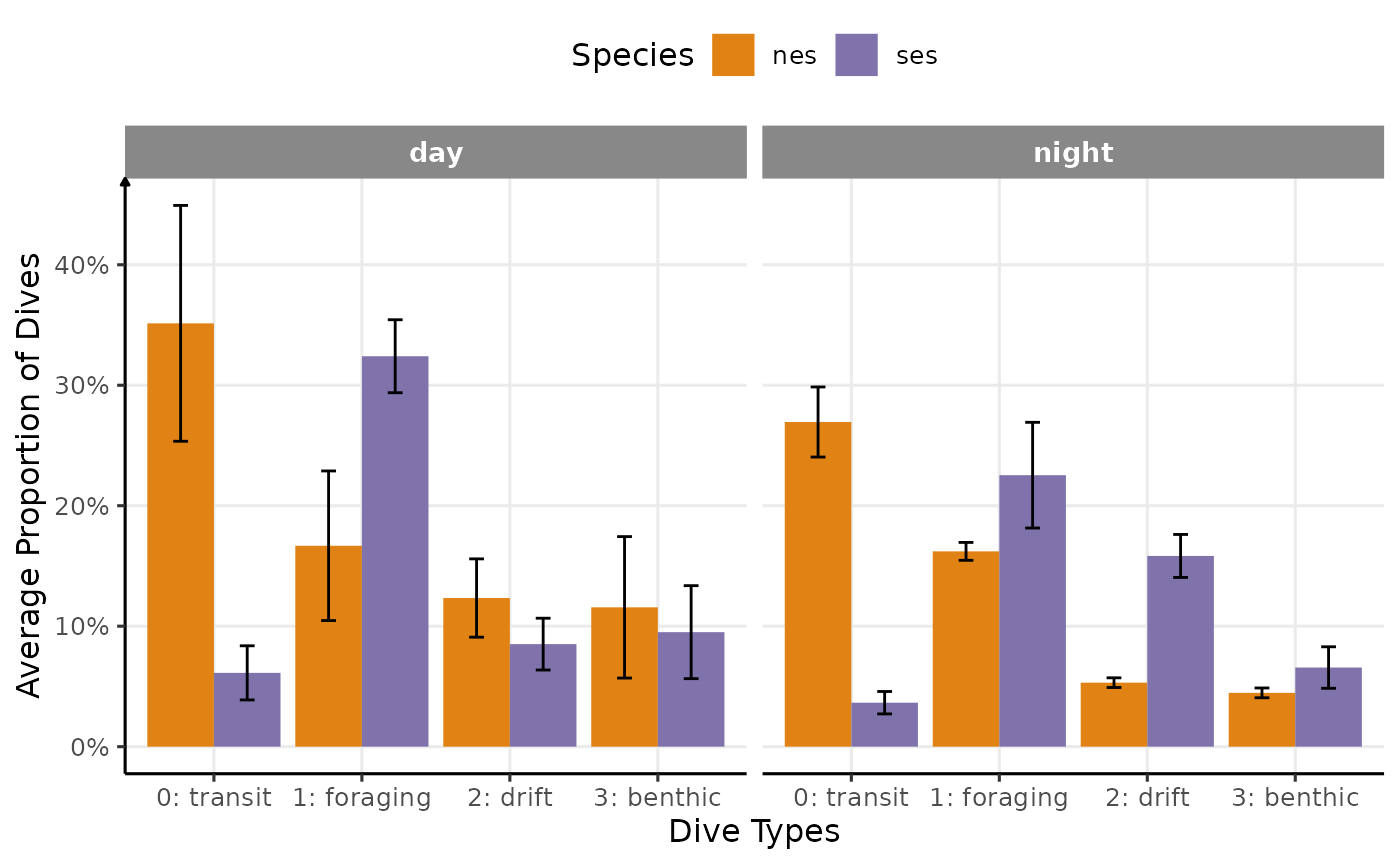
Proportion of dives by divetype, phases of the day and species (V1)
# plot
ggplot(dataPlot, aes(x = divetype, y = N, fill = phase)) +
geom_bar(stat = "identity", position = "dodge", color="grey30") +
geom_errorbar(aes(ymin=N-N_sd, ymax=N+N_sd), width=.2,
position=position_dodge(.9)) +
scale_y_continuous(labels = label_percent()) +
facet_grid(.~sp) +
theme_jjo() +
# day first, and night
scale_fill_manual(values = c("white", "grey")) +
labs(x = "Dive Types", y = "Average Proportion of Dives") +
theme(legend.position = "top",
axis.line.x = element_line(arrow = NULL))
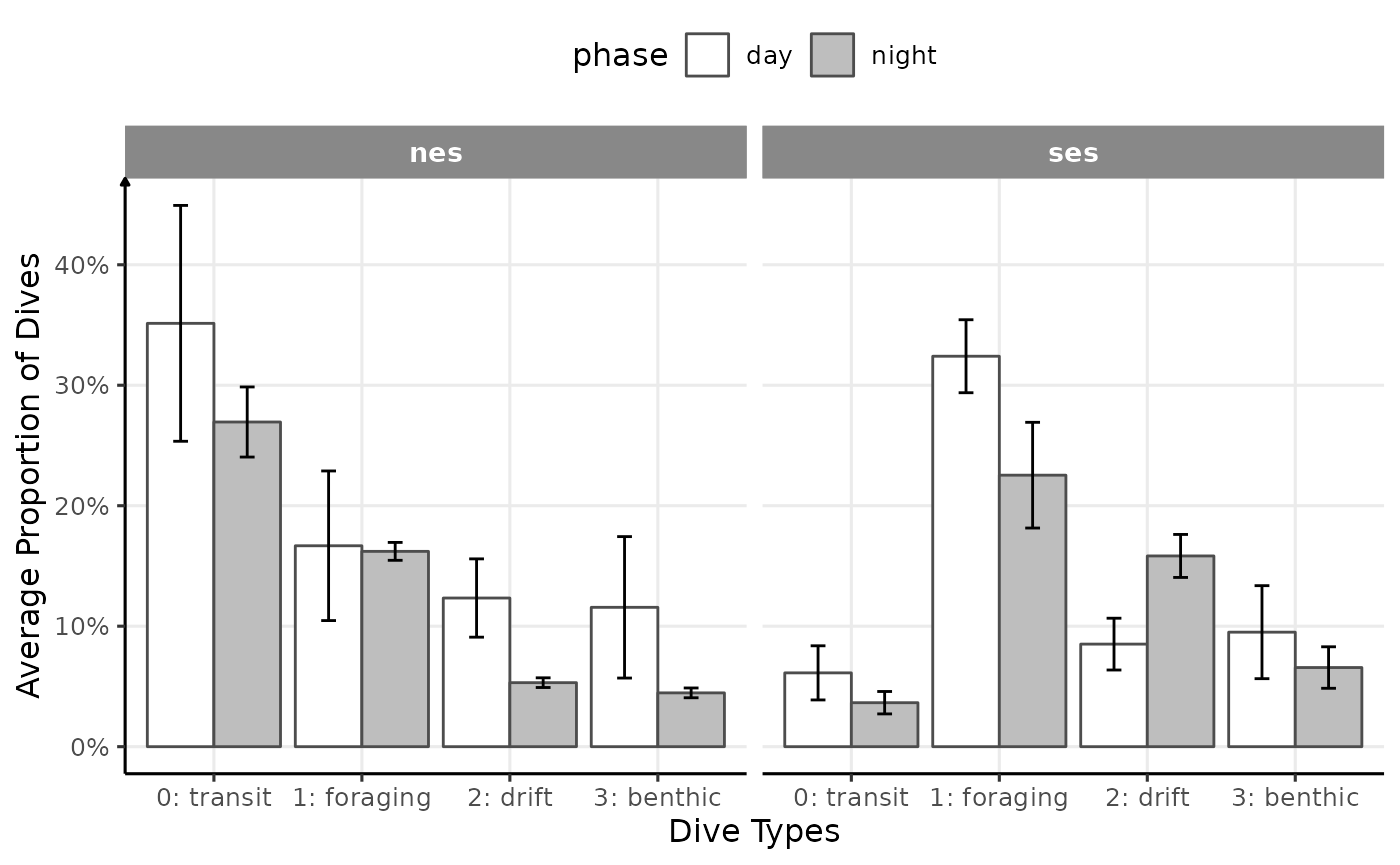
Proportion of dives by divetype, phases of the day and species (V2)
# plot
ggplot(copy(dataPlot)[sp=="nes",N:=-(1*N)], aes(x = divetype, y = N, fill = phase)) +
geom_bar(stat = "identity", position = "dodge", color="grey30") +
scale_y_continuous(labels = function(x) {percent(abs(x), 1)}) +
geom_errorbar(aes(ymin=N-N_sd, ymax=N+N_sd), width=.2,
position=position_dodge(.9)) +
coord_flip() +
facet_grid(.~sp, scales = "free_x") +
theme_jjo() +
# day first, and night
scale_fill_manual(values = c("white", "grey")) +
labs(x = "Dive Types", y = "Proportion of Dives") +
theme(legend.position = "top",
axis.line = element_line(arrow = NULL))
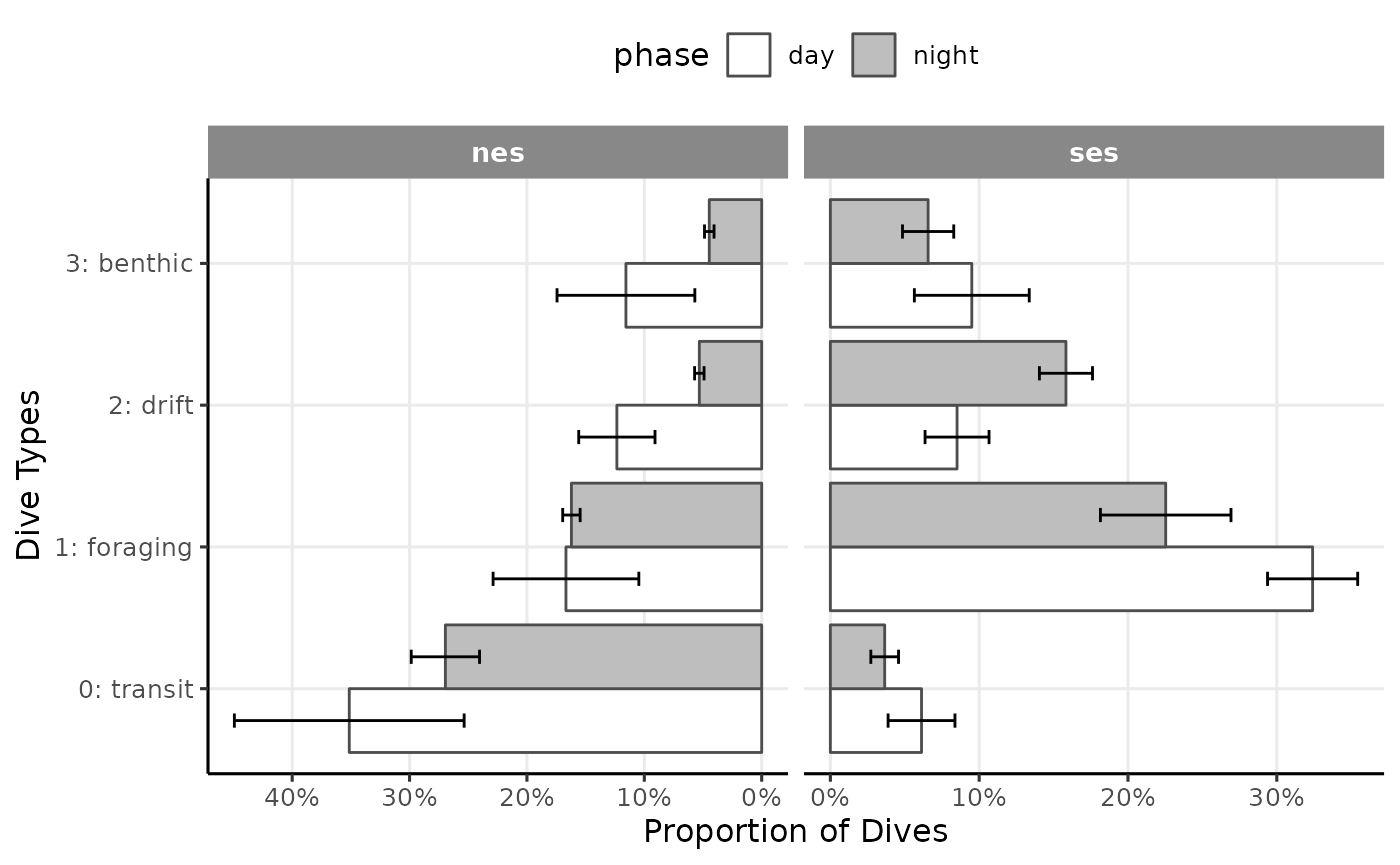
Proportion of dives by divetype, phases of the day and species (V3)
Max Depth
Let’s try to recreate the same plots Grecian, et al. (2022) did to compare the evolution of some behavioral parameters across time between nes and ses.
plot_comp(data_comp, "maxdepth", nb_days = 200, cols = "phase") +
labs(
x = "# days since departure",
y = "Maximum Depth (m)",
colour = "Species",
fill = "Species"
) +
scale_y_reverse() +
theme_jjo() +
theme(legend.position = "top")
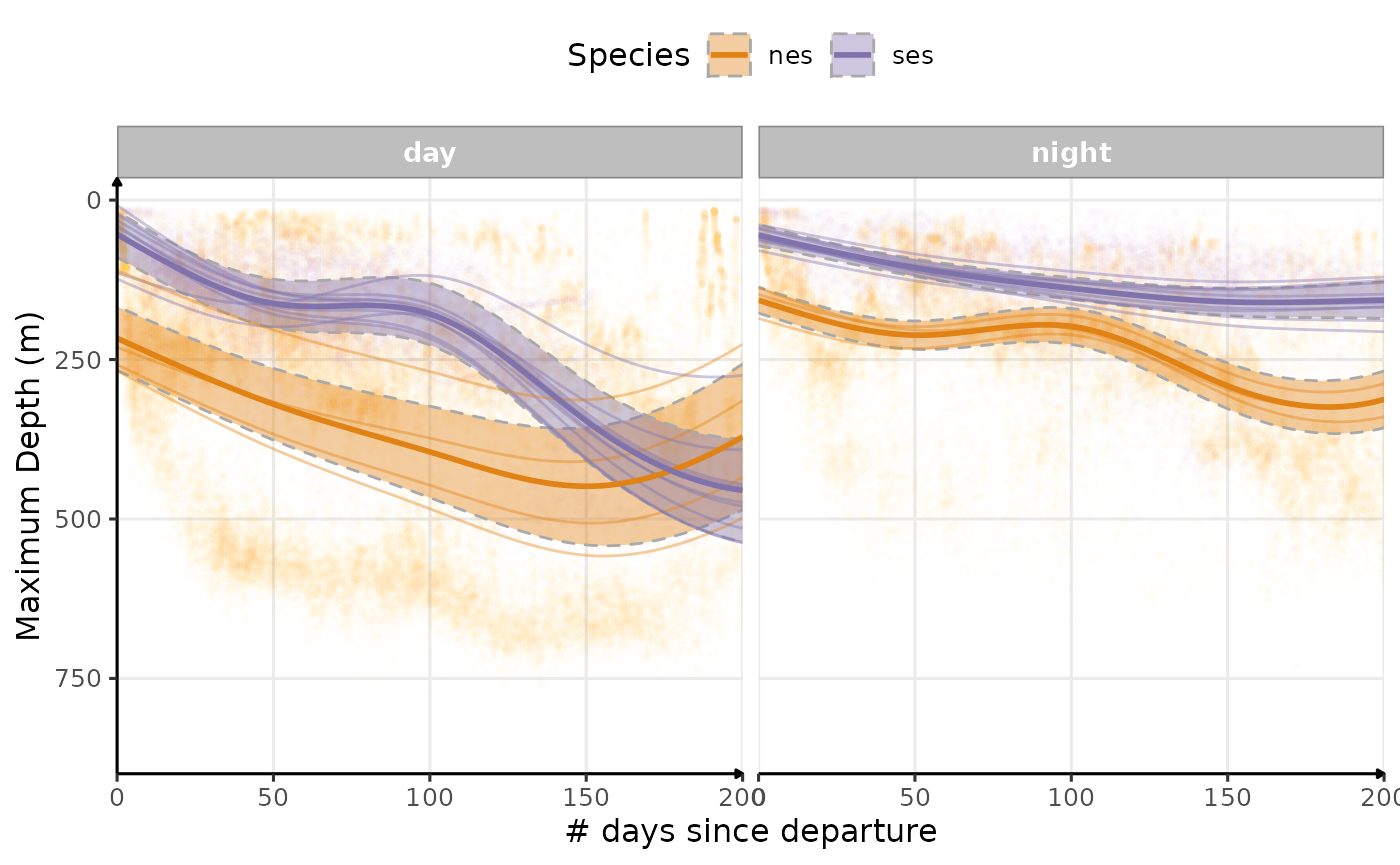
Estimated temporal changes in maximum depth (m) according to phases of the day
Since there is still this bimodality in the distribution of the
maximumm depth during the day for nes
, we splited by
divetype.
plot_comp(data_comp,
"maxdepth",
nb_days = 200,
cols = "phase",
rows = "divetype",
scales = "free_y") +
labs(
x = "# days since departure",
y = "Maximum Depth (m)",
colour = "Species",
fill = "Species"
) +
scale_y_reverse() +
theme_jjo() +
theme(legend.position = "top")
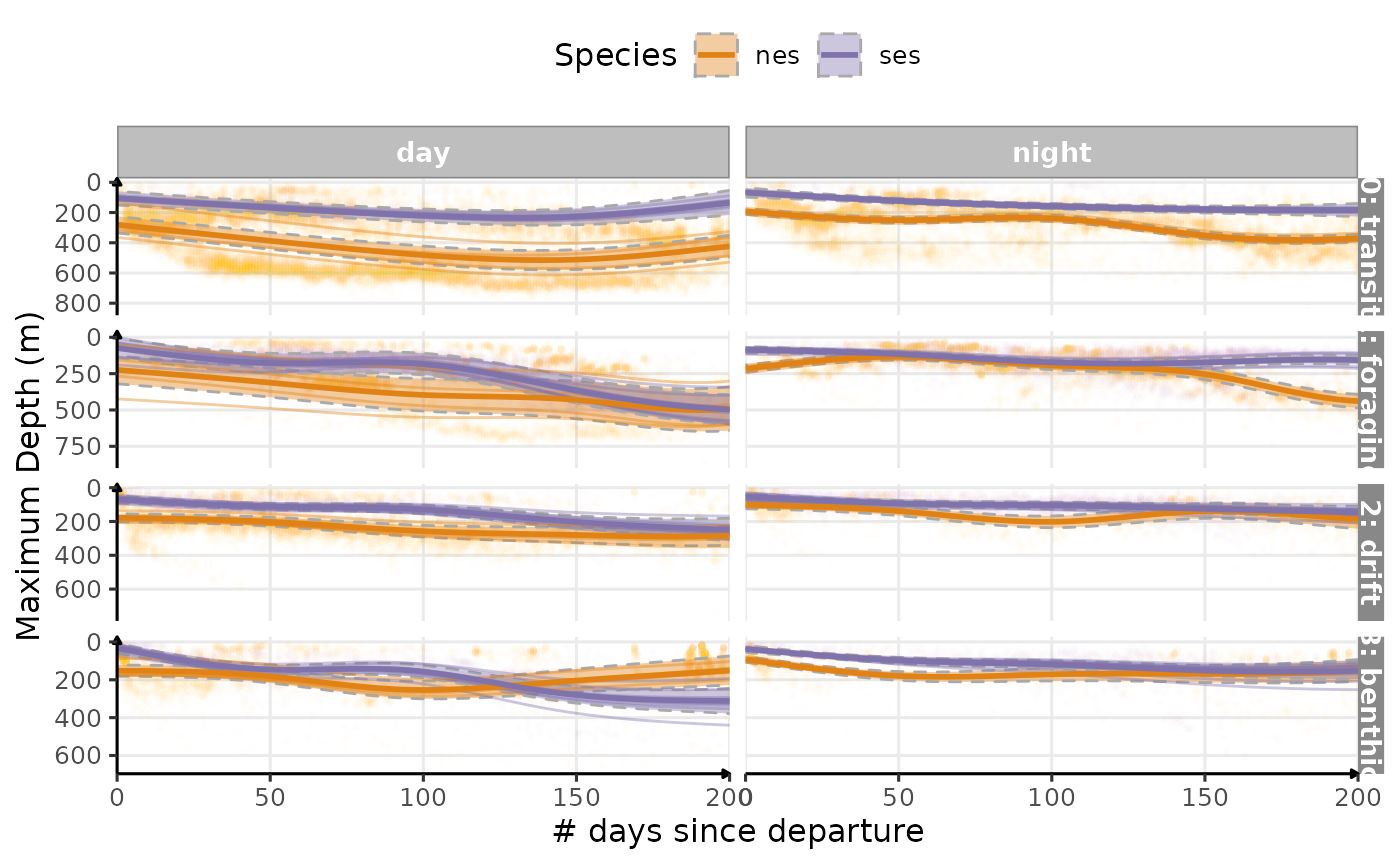
Estimated temporal changes in maximum depth (m) according to phases of the day and dive types (V1)
Still a bimodality in
day
forses
in transit dives…
plot_comp(data_comp,
"maxdepth",
nb_days = 200,
cols = "phase",
rows = "divetype",
scales = "free_y",
point = FALSE) +
labs(
x = "# days since departure",
y = "Maximum Depth (m)",
colour = "Species",
fill = "Species"
) +
scale_y_reverse() +
theme_jjo() +
theme(legend.position = "top")
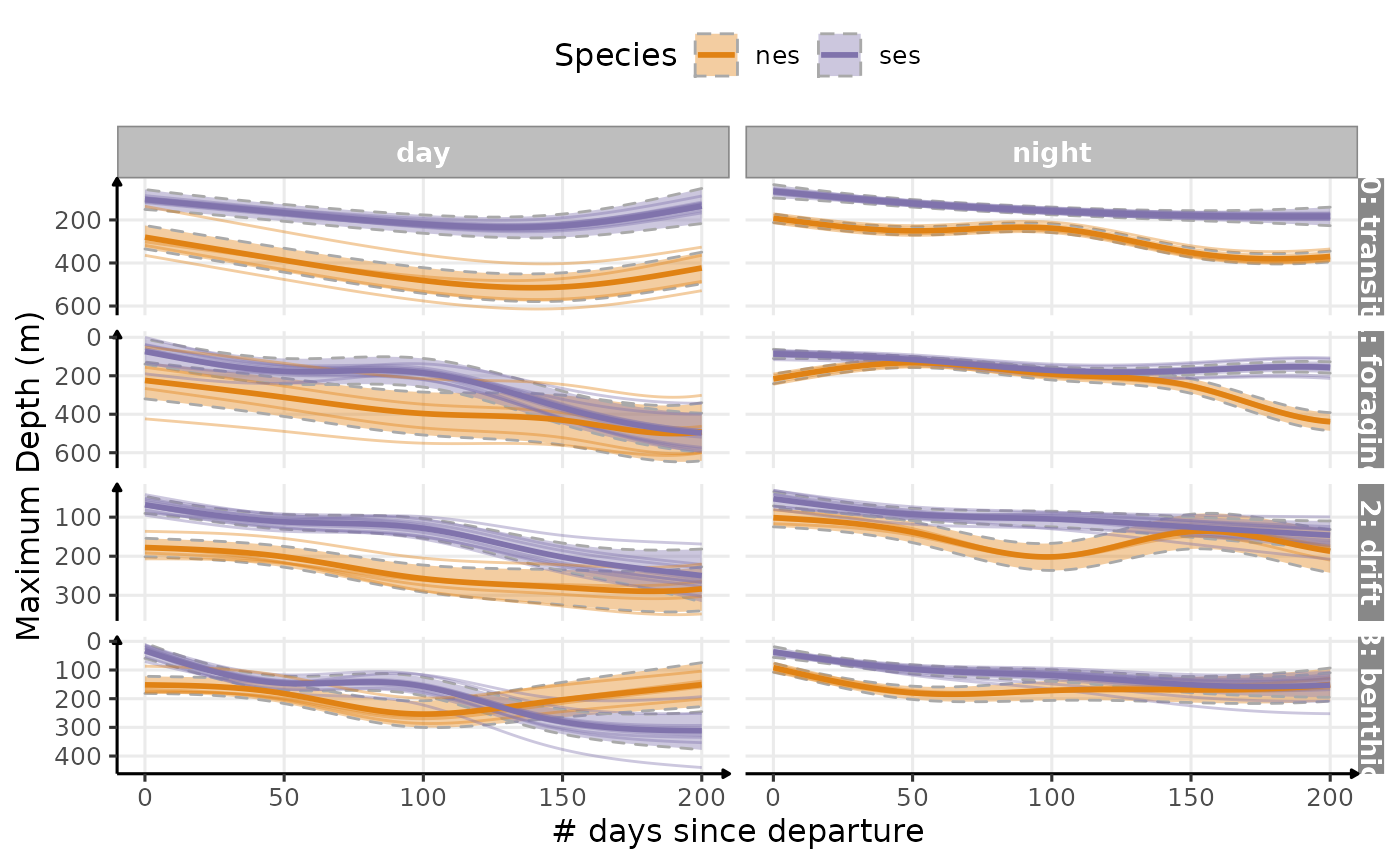
Estimated temporal changes in maximum depth (m) according to phases of the day and dive types (V2)
plot_comp(data_comp,
"maxdepth",
group_to_compare = "divetype",
nb_days = 200,
cols = "sp",
ribbon = T,
point = F,
scales = "free_y") +
labs(
x = "# days since departure",
y = "Maximum Depth (m)",
colour = "Species",
fill = "Species"
) +
scale_y_reverse() +
theme_jjo() +
theme(legend.position = "top")
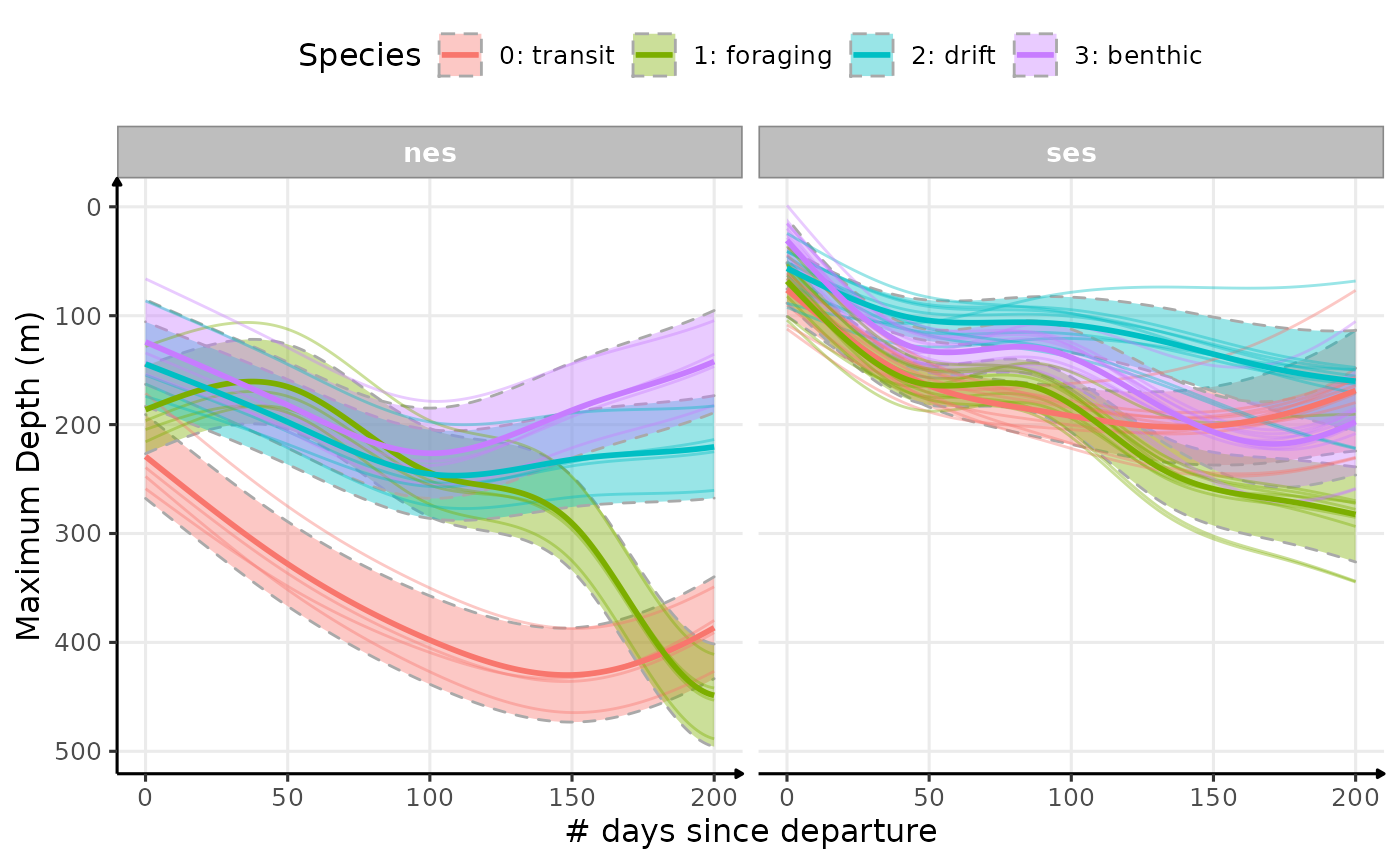
Estimated temporal changes in maximum depth (m) according to phases of the day and dive types (V3)
Dive Duration
plot_comp(data_comp, "dduration", nb_days = 200, cols = "phase") +
labs(
x = "# days since departure",
y = "Dive Duration (s)",
colour = "Species",
fill = "Species"
) +
theme_jjo() +
theme(legend.position = "top")
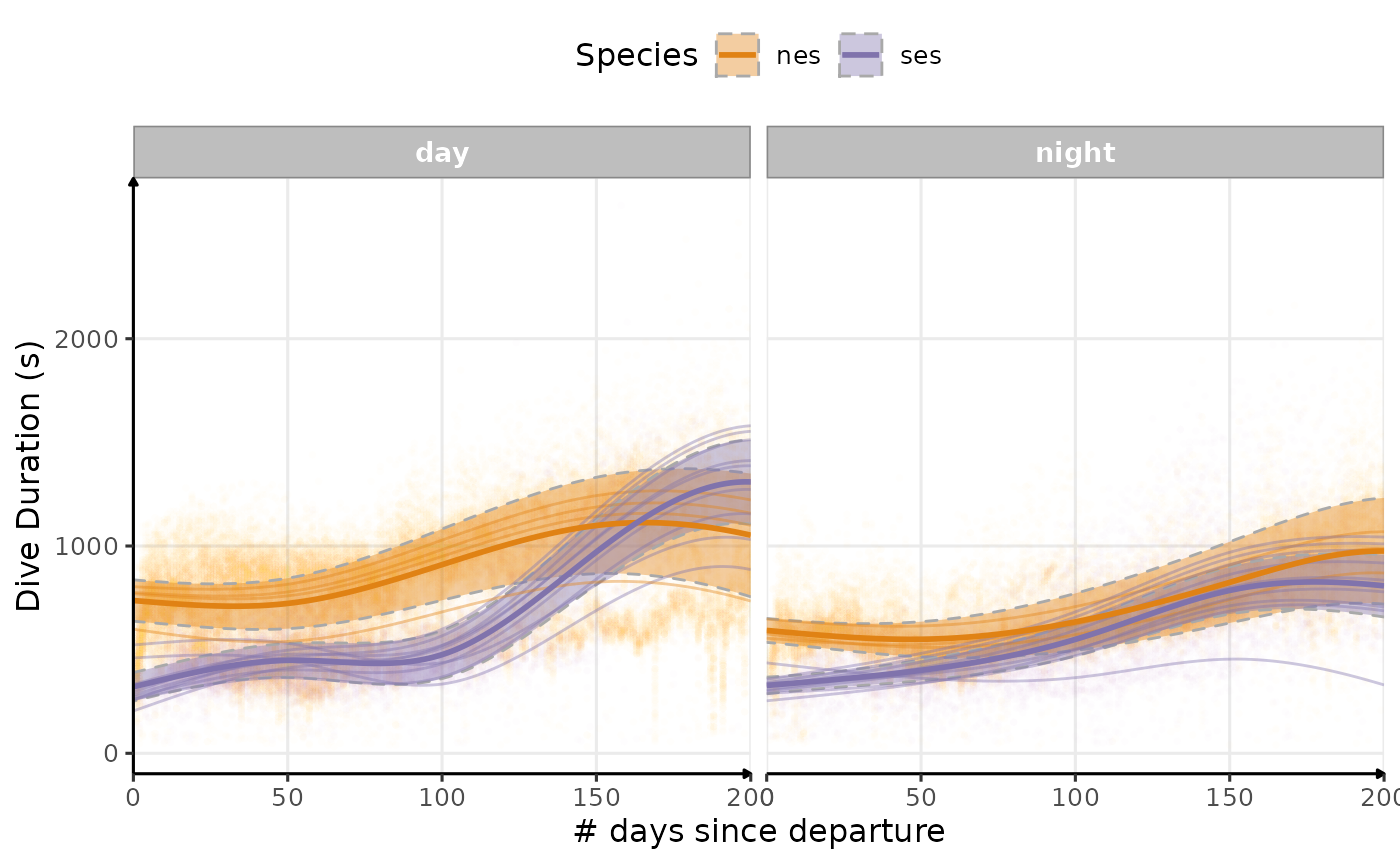
Estimated temporal changes in dive duration (s) according to phases of the day
plot_comp(data_comp,
"dduration",
nb_days = 200,
cols = "phase",
rows = "divetype",
scales = "free_y") +
labs(
x = "# days since departure",
y = "Dive Duration (s)",
colour = "Species",
fill = "Species"
) +
theme_jjo() +
theme(legend.position = "top")
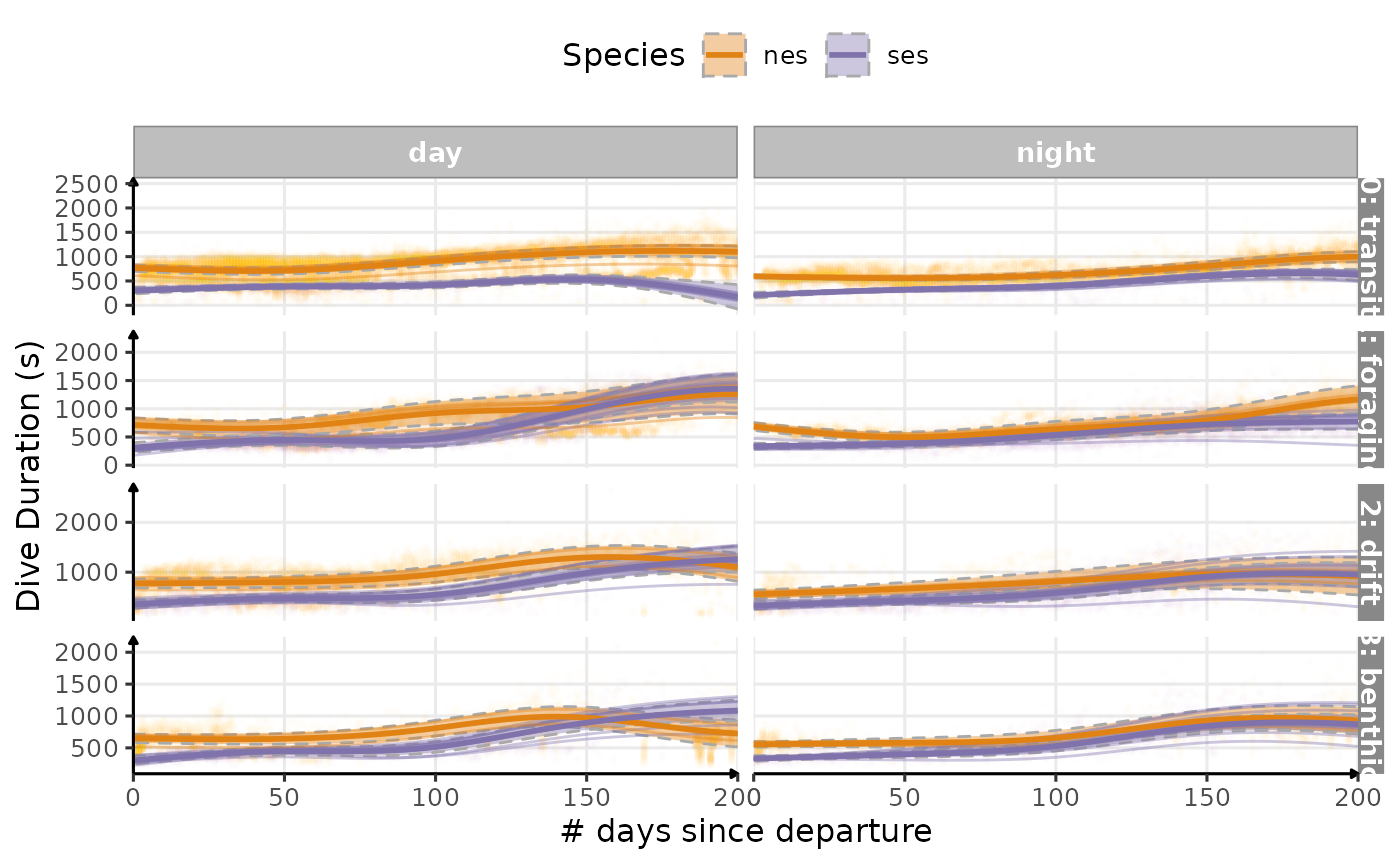
Estimated temporal changes in dive duration (s) according to phases of the day and dive types (V1)
plot_comp(data_comp,
"dduration",
group_to_compare = "divetype",
nb_days = 200,
cols = "sp",
ribbon = T,
point = F,
scales = "free_y",
k = 7) +
labs(
x = "# days since departure",
y = "Dive Duration (s)",
colour = "Species",
fill = "Species"
) +
theme_jjo() +
theme(legend.position = "top")
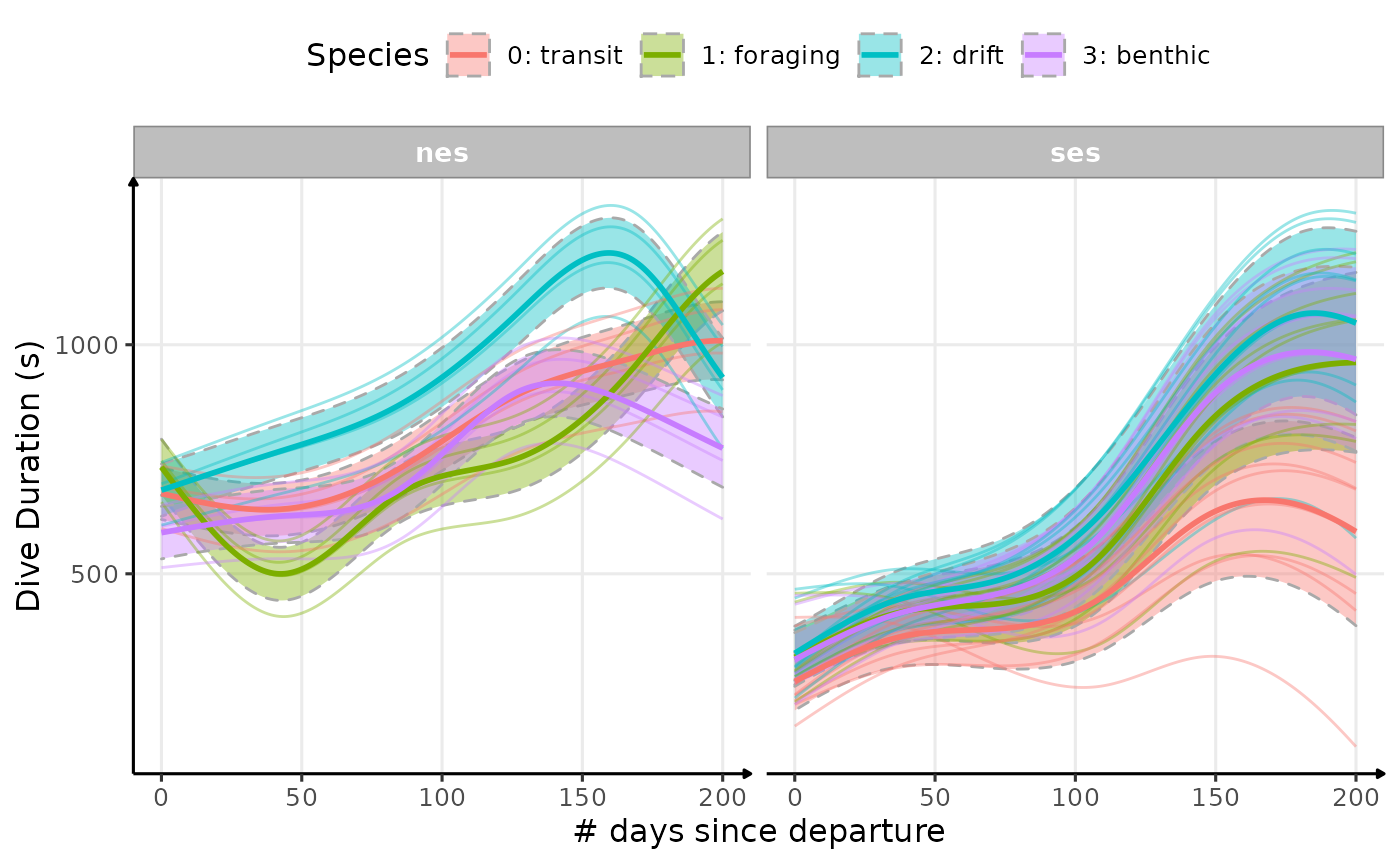
Estimated temporal changes in dive duration (s) according to phases of the day and dive types (V2)
“bADL”
# data nes
days_to_keep_nes = data_comp[sp=="nes",
.(nb_dives = .N),
by = .(.id, day_departure)] %>%
.[nb_dives >= 50,]
# keep only those days
data_nes_complete_day = merge(data_comp[sp == "nes",],
days_to_keep_nes,
by = c(".id", "day_departure"))
# data ses
days_to_keep_ses = data_comp[sp=="ses",
.(nb_dives = .N),
by = .(.id, day_departure)] %>%
.[nb_dives >= 8,]
# keep only those days
data_ses_complete_day = merge(data_comp[sp == "ses",],
days_to_keep_ses,
by = c(".id", "day_departure"))
# data plot
dataPlot = rbind(data_nes_complete_day[divetype=="1: foraging",
.(badl = quantile(dduration, 0.95)),
by = .(.id, day_departure, sp)],
data_ses_complete_day[divetype=="1: foraging",
.(badl = quantile(dduration, 0.95)),
by = .(.id, day_departure, sp)])
# comparative plot
plot_comp(dataPlot, "badl", nb_days = 200, alpha_point = .1) +
labs(
x = "# days since departure",
y = "bADL (s)",
col = "Species",
fill = "Species"
) +
theme_jjo()
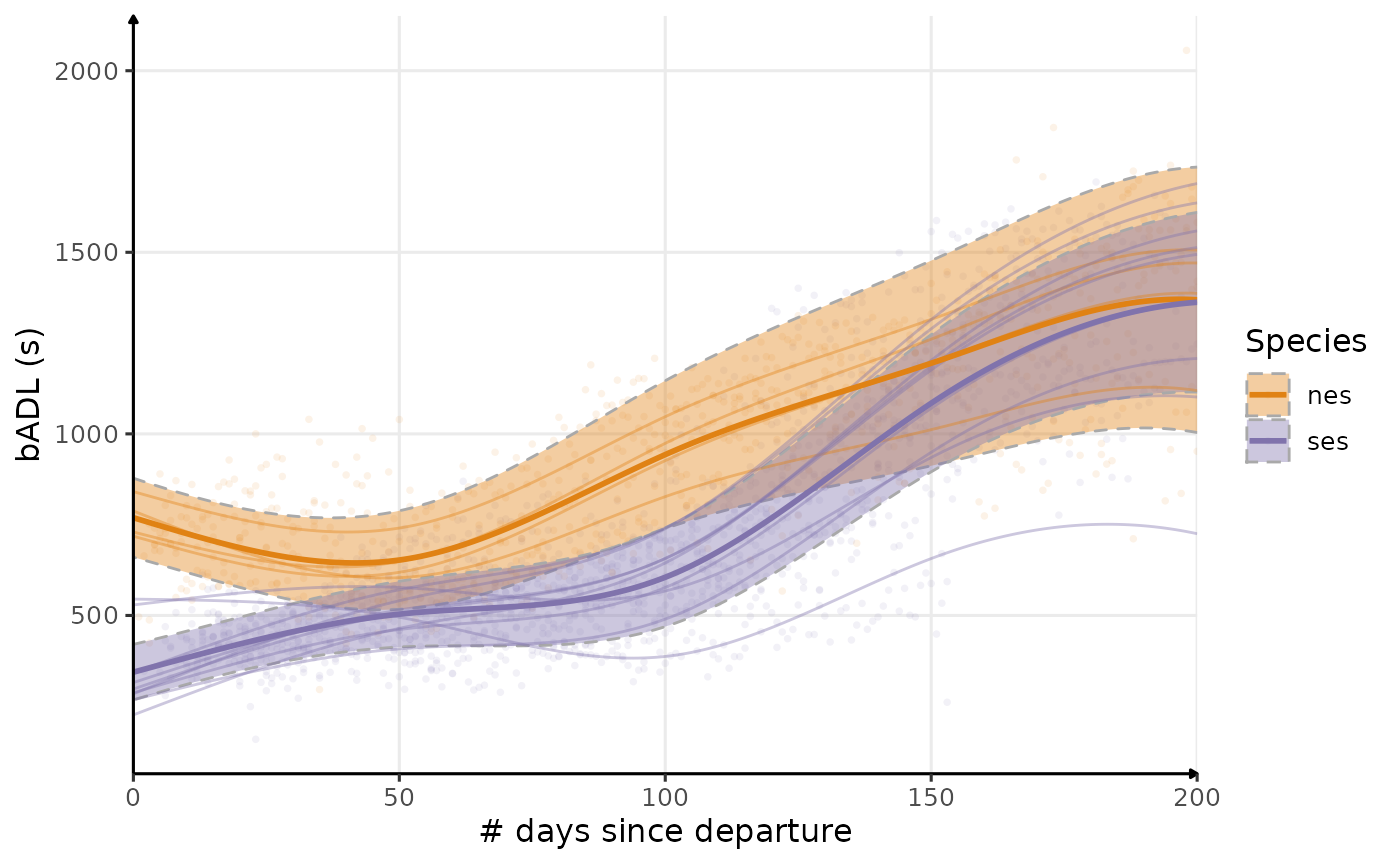
Estimated temporal changes in bADL (s)
Drift Rate
# calculate drift rate per day, id and sp
dataPlot = data_comp[divetype == "2: drift",
.(driftrate = quantile(driftrate, 0.5)),
by = .(day_departure, .id, sp)
]
# comparative plot
plot_comp(dataPlot, "driftrate", nb_days = 200, alpha_point = .1) +
labs(
x = "# days since departure",
y = "Drift Rate (m/s)",
title = "Day",
col = "Species",
fill = "Species"
) +
theme_jjo() +
theme(legend.position = "bottom")
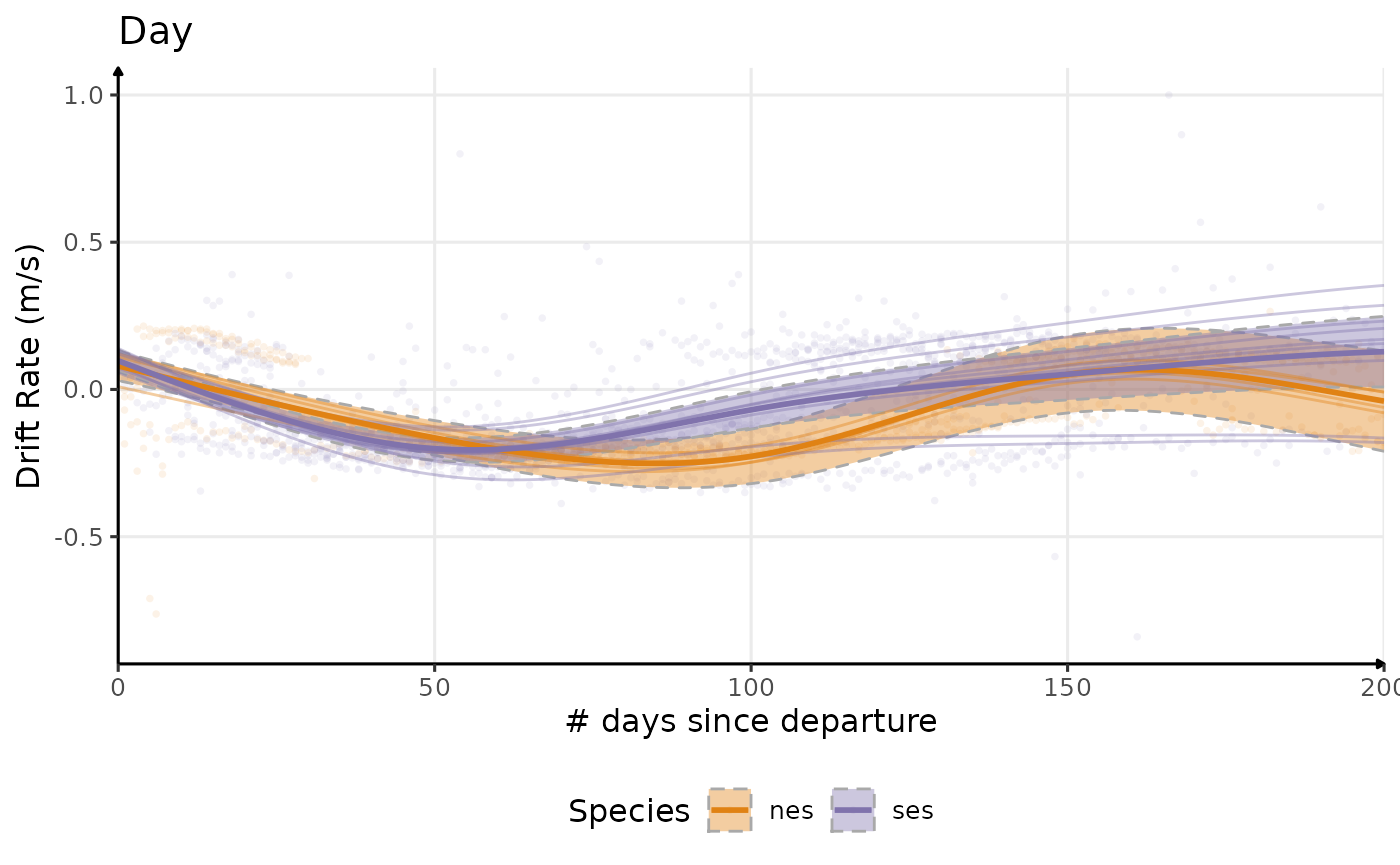
Estimated temporal changes in drift rate (m/s)
# comparative plot
plot_comp(dataPlot, "driftrate", nb_days = 200, alpha_point = .1, point = F) +
labs(
x = "# days since departure",
y = "Drift Rate (m/s)",
title = "Day",
col = "Species",
fill = "Species"
) +
theme_jjo() +
theme(legend.position = "bottom")
Maxdepth Bimodality Investigation
It’s weird that there is still a bimodality in the
maxdepth
’s distribution for northern elephant seal, even after splitting by phases of the day.
NES
To investigate why is that, let’s try several representation of this
data for the individual 2018070
(nes).
# let's pick an individual
data_test <- data_comp[.id == "ind_2018070", ]
# first we average `lightatsurf` by individuals, day since departure and hour
dataPlot <- data_test[, .(lightatsurf = median(lightatsurf, na.rm = T),
phase = first(phase)),
by = .(.id,
day_departure,
date = as.Date(date),
hour)]
# then we choose the variable to represent
i <- "maxdepth"
ggplot(data = melt(data_test[, .(.id, date, get(i), phase)],
id.vars = c(".id",
"date",
"phase")),
aes(x = as.Date(date),
y = value,
col = phase)) +
geom_point(alpha = 1 / 10,
size = .5) +
facet_wrap(. ~ .id, scales = "free") +
scale_x_date(date_labels = "%m/%Y") +
labs(x = "Date", y = i) +
scale_y_reverse() +
theme_jjo() +
theme(axis.text.x = element_text(angle = 45, hjust = 1),
legend.position = "bottom") +
guides(colour = guide_legend(override.aes = list(size = 7,
alpha = 1)))
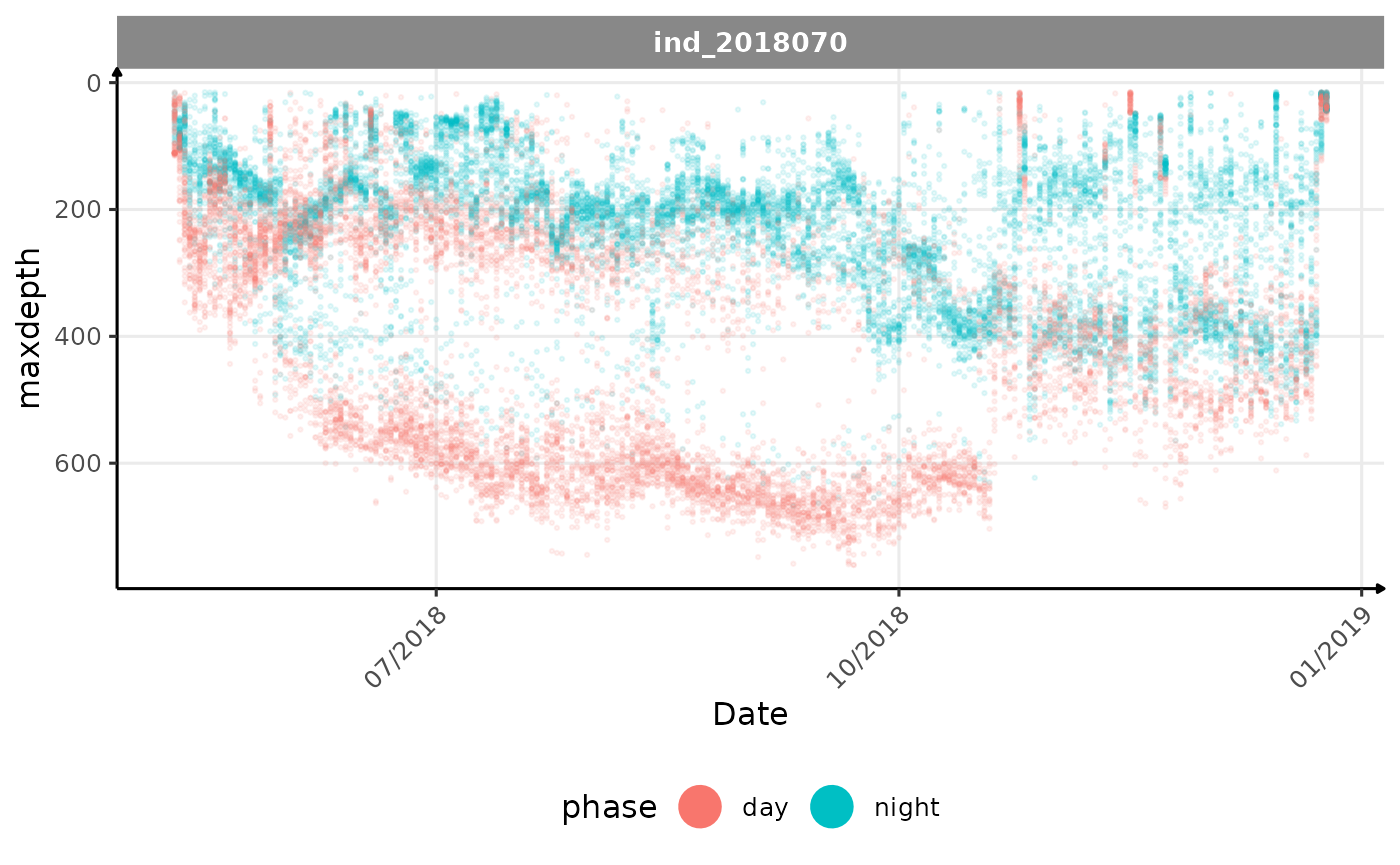
Evolution of the maximum depth reached across time for the individual 2018070
For this individual we can see that there are still a lot of shallow
dives during the day. I first thought it was because this individual
would probably have started reaching shallow water earlier in the day,
at dusk, rather than waiting for complete darkness. To test this
hypothesis, I tried to visualize the maxdepth
over an
entire day throughout the trip.
# this is art!
htmltools::tagList(
plot_ly() %>%
add_trace(
data = data_test,
x = ~hour,
y = ~day_departure,
z = ~maxdepth,
marker = list(
size = 1,
opacity = 0.5
),
mode = "markers",
type = "scatter3d",
text = ~divenumber,
hovertemplate = paste(
"Time: %{x:} h<br>",
"Since departure: %{y:} days<br>",
"Max Depth: %{z:} m<br>",
"Dive Number: %{text:}"
),
showlegend = FALSE
) %>%
add_trace(
x = ~hour,
y = ~day_departure,
z = ~ (lightatsurf / 200) * 20,
intensity = ~ lightatsurf / 200,
data = dataPlot,
type = "mesh3d",
hovertemplate = paste(
"Time: %{x:} h<br>",
"Since departure: %{y:} days<br>",
"Light Level: %{z:} ?"
)
) %>%
layout(
scene = list(
zaxis = list(title = "Maximum depth (m)",
autorange="reversed"),
yaxis = list(title = "# days since departure"),
xaxis = list(title = "Hour")
),
legend = list(itemsizing = "constant")
) %>%
hide_colorbar() %>%
config(modeBarButtons = list(list("toImage"),
# list("editInChartStudio"),
list("resetViews"),
list("pan3d"),
list("orbitRotation"),
list("tableRotation")))
)
We can see the bimodality of maxdepth
within a day is
not related to the time of the day, since swallow and deep dives might
both occurred at the same time in the day. Let’s see what happen if we
color dives with divetype
.
# this is art!
htmltools::tagList(
plot_ly() %>%
add_trace(
data = data_test,
x = ~hour,
y = ~day_departure,
z = ~maxdepth,
color = ~divetype,
marker = list(
size = 1,
opacity = 0.5
),
mode = "markers",
type = "scatter3d",
text = ~divenumber,
hovertemplate = paste(
"Time: %{x:} h<br>",
"Since departure: %{y:} days<br>",
"Max Depth: %{z:} m<br>",
"Dive Number: %{text:}"
)
) %>%
add_trace(
x = ~hour,
y = ~day_departure,
z = ~ (lightatsurf / 200) * 20,
intensity = ~ lightatsurf / 200,
data = dataPlot,
type = "mesh3d",
showlegend = FALSE,
hovertemplate = paste(
"Time: %{x:} h<br>",
"Since departure: %{y:} days<br>",
"Light Level: %{z:} ?"
)
) %>%
layout(
scene = list(
zaxis = list(title = "Maximum depth (m)",
autorange="reversed"),
yaxis = list(title = "# days since departure"),
xaxis = list(title = "Hour")
),
legend = list(itemsizing = "constant")
) %>%
hide_colorbar() %>%
config(modeBarButtons = list(list("toImage"),
# list("editInChartStudio"),
list("resetViews"),
list("pan3d"),
list("orbitRotation"),
list("tableRotation")))
)
Well it seems that the bimodality observed in the distribution of
maxdepth
is mostly explained by divetype
, with
transit dives occurring at deeper dives that foraging
and drift dives (at least for northern elephant seal). We can
actually look at the same result with a simple 2D plot:
ggplot(
data = melt(data_test[, .(.id, date, get(i), divetype)],
id.vars = c(
".id",
"date",
"divetype"
)
),
aes(
x = as.Date(date),
y = value,
col = divetype
)
) +
geom_point(
alpha = 1 / 10,
size = .5
) +
facet_wrap(. ~ .id, scales = "free") +
scale_x_date(date_labels = "%m/%Y") +
labs(x = "Date", y = i) +
theme_jjo() +
scale_y_reverse() +
theme(
axis.text.x = element_text(angle = 45, hjust = 1),
legend.position = "bottom"
) +
guides(colour = guide_legend(override.aes = list(
size = 7,
alpha = 1
)))
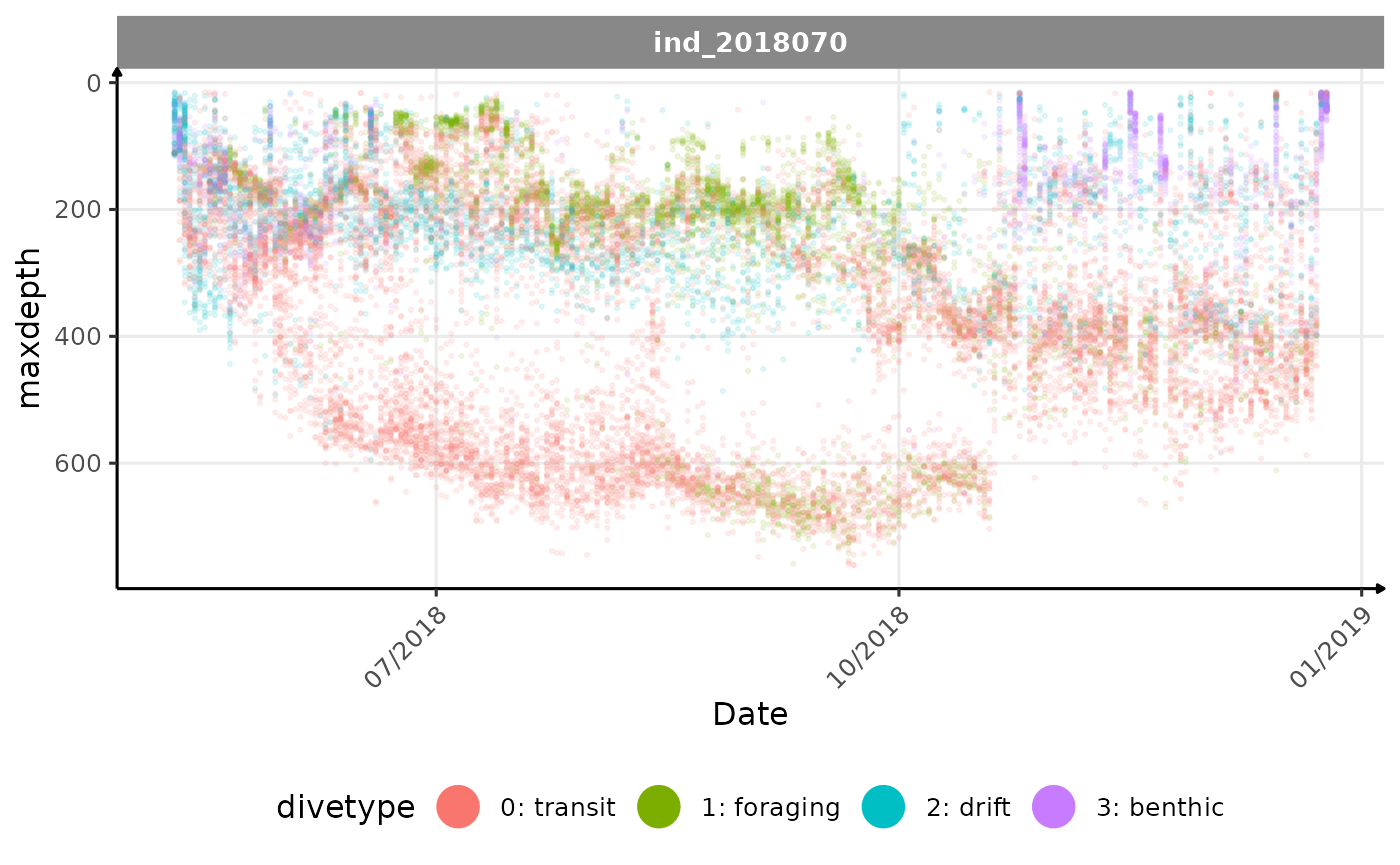
Kind of the same graph, but without looking at the hour of the day
And in bonus, we can add the bathymetry to the 3D plot.
htmltools::tagList(
plot_ly() %>%
add_trace(
data = data_test,
x = ~hour,
y = ~day_departure,
z = ~maxdepth,
color = ~divetype,
marker = list(
size = 1,
opacity = 0.5
),
mode = "markers",
type = "scatter3d",
text = ~divenumber,
hovertemplate = paste(
"Time: %{x:} h<br>",
"Since departure: %{y:} days<br>",
"Max Depth: %{z:} m<br>",
"Dive Number: %{text:}"
),
legendgroup = 'group1',
legendgrouptitle = list(text="Dive Types")
) %>%
add_trace(
x = ~hour,
y = ~day_departure,
z = ~ (lightatsurf / 200) * 20,
intensity = ~lightatsurf,
data = dataPlot,
type = "mesh3d",
legendgroup = "group2",
name = "Light Level",
showlegend = TRUE,
hovertemplate = paste(
"Time: %{x:} h<br>",
"Since departure: %{y:} days<br>",
"Light Level: %{z:} ?"
)
) %>%
hide_colorbar() %>%
add_trace(
x = ~hour,
y = ~day_departure,
z = ~abs(bathy),
color = "brown",
name = "Bathymetry",
data = data_test,
type = "mesh3d",
showlegend = TRUE,
legendgroup = 'group2',
legendgrouptitle = list(text="Other layers"),
hovertemplate = paste(
"Bathymetry: %{z:} m"
)
) %>%
layout(
scene = list(
zaxis = list(title = "Maximum depth (m)",
autorange="reversed"),
yaxis = list(title = "# days since departure"),
xaxis = list(title = "Hour")
),
legend = list(itemsizing = "constant",
groupclick = "toggleitem")
) %>%
config(modeBarButtons = list(list("toImage"),
# list("editInChartStudio"),
list("resetViews"),
list("pan3d"),
list("orbitRotation"),
list("tableRotation")))
)
SES
Let’s do the same analysis on the individual 140059
(ses).
# let's pick an individual
data_test <- data_comp[.id == "ind_140059",]
# first we average `lightatsurf` by individuals, day since departure and hour
dataPlot <- data_test[, .(lightatsurf = median(lightatsurf, na.rm = T),
phase = first(phase)),
by = .(.id,
day_departure,
date = as.Date(date),
hour)]
# then we choose the variable to represent
i <- "maxdepth"
ggplot(data = melt(data_test[, .(.id, date, get(i), phase)],
id.vars = c(".id",
"date",
"phase")),
aes(x = as.Date(date),
y = value,
col = phase)) +
geom_point(alpha = 1 / 5,
size = .5) +
facet_wrap(. ~ .id, scales = "free") +
scale_x_date(date_labels = "%m/%Y") +
labs(x = "Date", y = i) +
scale_y_reverse() +
theme_jjo() +
theme(axis.text.x = element_text(angle = 45, hjust = 1),
legend.position = "bottom") +
guides(colour = guide_legend(override.aes = list(size = 7,
alpha = 1)))
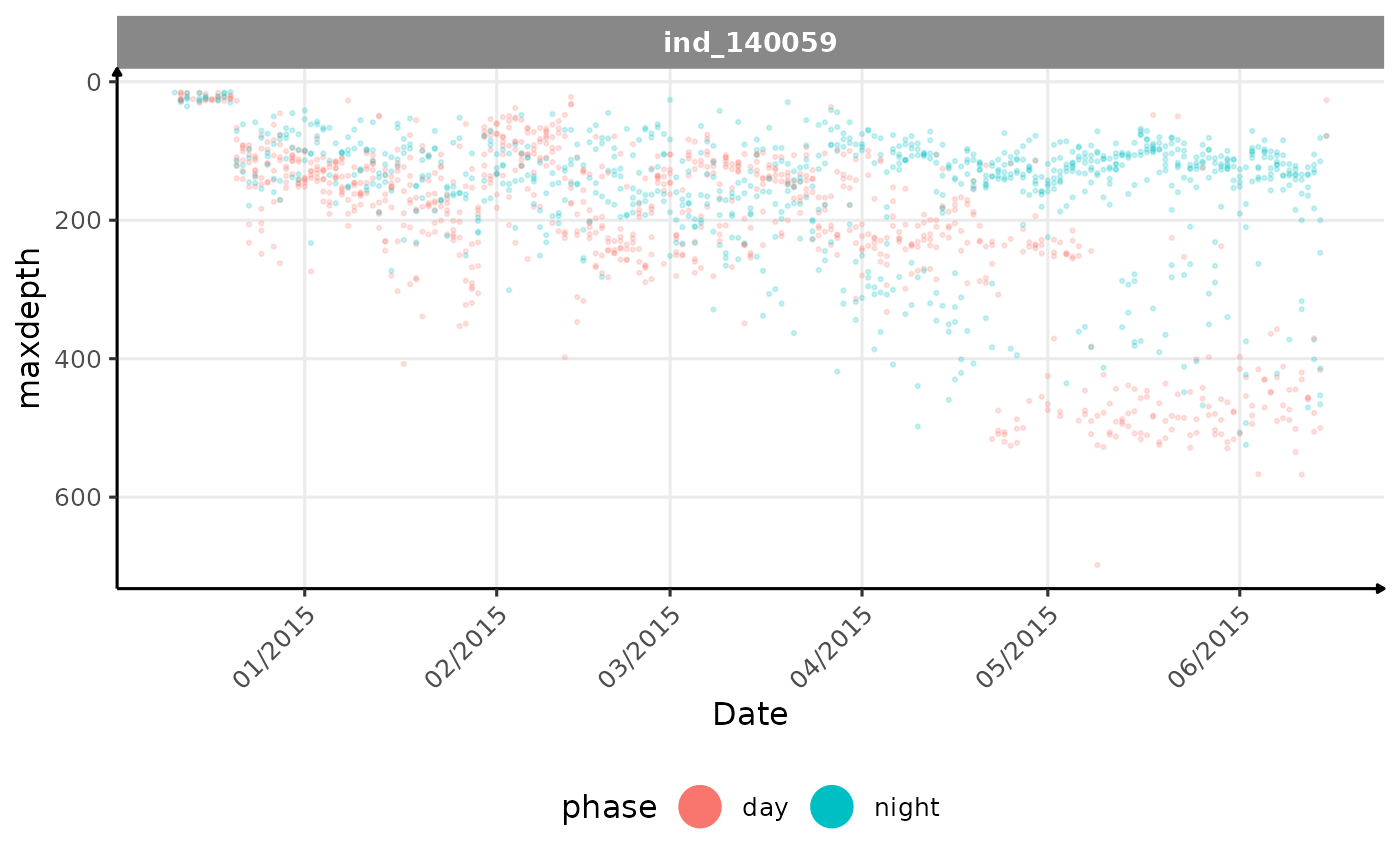
Evolution of the maximum depth reached across time for the individual 140059
ggplot(data = melt(data_test[, .(.id, date, get(i), divetype)],
id.vars = c(".id",
"date",
"divetype")),
aes(x = as.Date(date),
y = value,
col = divetype)) +
geom_point(alpha = 1 / 5,
size = .5) +
facet_wrap(. ~ .id, scales = "free") +
scale_x_date(date_labels = "%m/%Y") +
labs(x = "Date", y = i) +
scale_y_reverse() +
theme_jjo() +
theme(axis.text.x = element_text(angle = 45, hjust = 1),
legend.position = "bottom") +
guides(colour = guide_legend(override.aes = list(size = 7,
alpha = 1)))
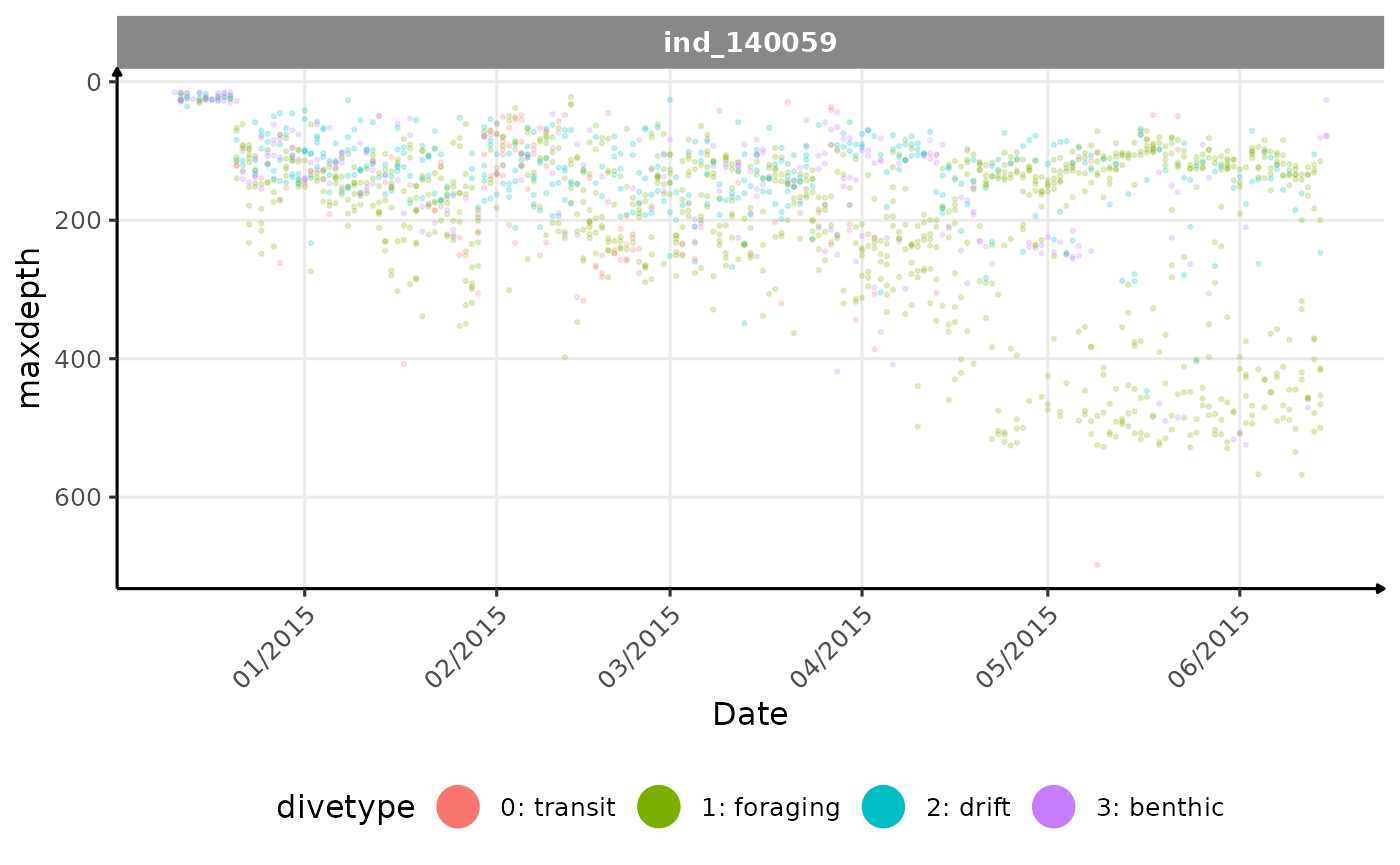
Evolution of the maximum depth reached across time for the individual 140059
For this individual we can see that until the half of its trip, there
is no distinction between day and night in terms of
maxdepth
. But After, he starts to dive deeper during the
day, and shallower during the night. Contrary to the previous nes
individual, there seems to be a clear pattern. Let’s look at the graph
in 3D:
htmltools::tagList(
plot_ly() %>%
add_trace(
data = data_test,
x = ~hour,
y = ~day_departure,
z = ~maxdepth,
color = ~divetype,
marker = list(
size = 1,
opacity = 0.8
),
mode = "markers",
type = "scatter3d",
text = ~divenumber,
hovertemplate = paste(
"Time: %{x:} h<br>",
"Since departure: %{y:} days<br>",
"Max Depth: %{z:} m<br>",
"Dive Number: %{text:}"
),
legendgroup = 'group1',
legendgrouptitle = list(text="Dive Types")
) %>%
add_trace(
x = ~hour,
y = ~day_departure,
z = ~ (hour * 0.1) + 20,
intensity = ~phase_bool,
data = dataPlot[][, phase_bool := fifelse(phase == "night", 0, 1)][],
type = "mesh3d",
name = "Phases of the day",
showlegend = TRUE,
legendgroup = 'group2',
legendgrouptitle = list(text="Other layers"),
hovertemplate = paste(
"Bathymetry: %{z:} m"
)
) %>%
add_trace(
x = ~hour,
y = ~day_departure,
z = ~abs(bathy),
color = "brown",
name = "Bathymetry",
data = data_test,
type = "mesh3d",
showlegend = TRUE,
legendgroup = 'group2',
legendgrouptitle = list(text="Other layers"),
hovertemplate = paste(
"Bathymetry: %{z:} m"
)
) %>%
hide_colorbar() %>%
layout(
scene = list(
zaxis = list(title = "Maximum depth (m)",
autorange = "reversed"),
yaxis = list(title = "# days since departure"),
xaxis = list(title = "Hour")
),
legend = list(itemsizing = "constant",
groupclick = "toggleitem")
)%>%
config(modeBarButtons = list(list("toImage"),
# list("editInChartStudio"),
list("resetViews"),
list("pan3d"),
list("orbitRotation"),
list("tableRotation")))
)