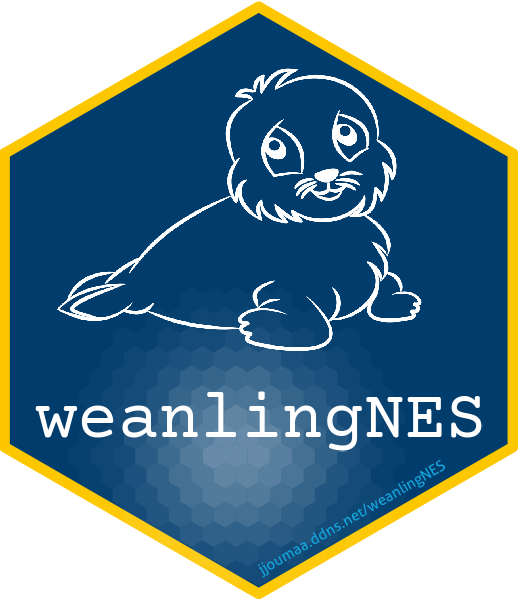
Data Consistency SES - 2014
Joffrey JOUMAA
October 21, 2022
data_consistency_ses.Rmd
Summary information
Let’s try to grab information regarding the data available for all individuals.
# summarize data
summary_data = rbindlist(lapply(list.dirs("../inst/extdata/cebc/", full.names = T, recursive = F), function(x){
# list files
files_data = list.dirs(x, full.names = F)
# data.table to store results
data.table(.id = tolower(last(strsplit(x, split = "/")[[1]])),
low_res = fifelse("DSA_BasseRes" %in% files_data, T, F),
high_res = fifelse("DSA_HauteRes" %in% files_data, T, F),
spot = fifelse("Spot" %in% files_data, T, F))
}))
# print
summary_data %>% sable(caption = "Summary information regarding datasets on southern elephant seals")
.id | low_res | high_res | spot |
---|---|---|---|
ind_140059 | TRUE | TRUE | TRUE |
ind_140060 | TRUE | TRUE | TRUE |
ind_140061 | TRUE | FALSE | TRUE |
ind_140062 | TRUE | TRUE | TRUE |
ind_140063 | TRUE | TRUE | TRUE |
ind_140064 | TRUE | FALSE | TRUE |
ind_140065 | TRUE | FALSE | TRUE |
ind_140066 | TRUE | FALSE | TRUE |
ind_140067 | TRUE | FALSE | TRUE |
ind_140068 | TRUE | TRUE | TRUE |
ind_140069 | TRUE | TRUE | TRUE |
ind_140070 | TRUE | FALSE | TRUE |
ind_140071 | TRUE | FALSE | TRUE |
ind_140072 | TRUE | TRUE | TRUE |
ind_140073 | TRUE | TRUE | TRUE |
ind_140074 | TRUE | FALSE | TRUE |
ind_140075 | TRUE | TRUE | TRUE |
ind_140076 | TRUE | FALSE | TRUE |
ind_140077 | TRUE | FALSE | TRUE |
ind_140078 | TRUE | FALSE | TRUE |
We have high resolution data (9 seals) for most of the seals that survived, but not all of them. I’m especially wondering why we do not have high resolution for
ind_14066
andind_14077
?
Consistency across *.wch
files
It seems for each high-resolution individuals, data have been split
into several files (...wch000...
,
...wch001...
, ...wch002...
, …). I’m wondering
why, and if we can append each files without having a gap in our
data.
# calculate start and end of each file
summary_files = rbindlist(lapply(list.dirs(
"../inst/extdata/cebc",
full.names = T,
recursive = F
), function(x) {
# list files
files_data = list.dirs(x, full.names = F)
# id of the seal
ind_name = last(strsplit(x, split = "/")[[1]])
# check for high resolution
if ("DSA_HauteRes" %in% files_data) {
# list csv files
list_files = list.files(paste0(x, "/DSA_HauteRes"),
pattern = "*-Archive.csv",
full.names = T)
# extract start and end date
summary_files_inter = rbindlist(lapply(list_files, function(y) {
# import first col => Time
df = fread(y, select = 1, fill = T)
start = df[1, 1][[1]]
end = df[.N, 1][[1]]
# export
return(data.table(
start_date = start,
end_date = end,
file = gsub(".*[.]([^.]+)[-].*", "\\1", y)
))
}))
# add id seals
summary_files_inter[, .id := tolower(ind_name)]
# return
return(summary_files_inter)
}
}))
# format into Posixt
summary_files[, `:=` (start_date = as.character(as.POSIXct(start_date, format = "%d/%M/%Y %T", tz = "GMT")),
end_date = as.character(as.POSIXct(end_date, format = "%d/%M/%Y %T", tz = "GMT")))]
# reformat the output
summary_files = dcast(summary_files, .id~file, value.var = c("start_date","end_date"))
# reorder the column
setcolorder(summary_files,
neworder = c(".id",
"start_date_wch000","end_date_wch000",
"start_date_wch001","end_date_wch001",
"start_date_wch002","end_date_wch002",
"start_date_wch003","end_date_wch003",
"start_date_wch004","end_date_wch004"))
# lets rename column
col_labs <- lapply(colnames(summary_files), function(x) {
if (x == ".id") {
return(".id")
} else {
ifelse(grepl("start", x), return("Start"), return("End"))
}
})
names(col_labs) = colnames(summary_files)
Here is the result:
# print
summary_files %>%
gt() %>%
tab_header(title = "Gap check within the data on the southern elephant seals") %>%
tab_spanner(label = "wch000", columns = matches("wch000")) %>%
tab_spanner(label = "wch001", columns = matches("wch001")) %>%
tab_spanner(label = "wch002", columns = matches("wch002")) %>%
tab_spanner(label = "wch003", columns = matches("wch003")) %>%
tab_spanner(label = "wch004", columns = matches("wch004")) %>%
cols_label(.list = col_labs) %>%
fmt_datetime(
columns = matches("date"),
date_style = 14,
time_style = 2
)
Gap check within the data on the southern elephant seals | ||||||||||
.id | wch000 | wch001 | wch002 | wch003 | wch004 | |||||
---|---|---|---|---|---|---|---|---|---|---|
Start | End | Start | End | Start | End | Start | End | Start | End | |
ind_140059 | 14/10/12 18:22 | 15/10/03 19:00 | 15/10/03 21:05 | 15/10/04 05:31 | 15/10/04 07:39 | 15/10/05 15:26 | 15/10/05 17:49 | 15/10/07 12:35 | 15/10/07 14:43 | 15/10/08 23:37 |
ind_140060 | 14/10/12 21:47 | 15/10/02 23:00 | 15/10/02 01:04 | 15/10/04 07:47 | 15/10/04 09:54 | 15/10/06 05:50 | 15/10/06 08:08 | 15/10/06 07:32 | NA | NA |
ind_140062 | 14/10/12 18:31 | 15/10/02 06:55 | 15/10/02 09:03 | 15/10/05 20:40 | 15/10/05 22:45 | 15/10/07 04:08 | 15/10/07 06:24 | 15/10/09 15:25 | 15/10/09 17:38 | 15/10/09 16:52 |
ind_140063 | 14/10/12 15:25 | 15/10/02 19:38 | 15/10/02 22:15 | 15/10/04 15:32 | 15/10/04 18:12 | 15/10/05 03:33 | NA | NA | NA | NA |
ind_140068 | 14/10/12 18:24 | 15/10/03 19:35 | 15/10/03 21:45 | 15/10/04 13:05 | 15/10/04 15:12 | 15/10/06 06:50 | 15/10/06 09:18 | 15/10/06 17:09 | NA | NA |
ind_140069 | 14/10/12 19:23 | 15/10/03 17:34 | 15/10/03 19:47 | 15/10/05 07:09 | 15/10/05 09:25 | 15/10/06 09:41 | 15/10/06 11:45 | 15/10/07 05:10 | NA | NA |
ind_140072 | 14/10/12 20:21 | 15/10/03 02:51 | 15/10/03 04:54 | 15/10/05 07:20 | 15/10/05 09:39 | 15/10/07 22:27 | 15/10/07 00:32 | 15/10/08 10:03 | 15/10/08 12:20 | 15/10/09 03:18 |
ind_140073 | 14/10/12 19:43 | 15/10/03 04:44 | 15/10/03 06:59 | 15/10/04 10:15 | 15/10/04 12:32 | 15/10/05 02:31 | NA | NA | NA | NA |
ind_140075 | 14/10/12 18:28 | 15/10/03 14:03 | 15/10/03 16:07 | 15/10/04 04:44 | NA | NA | NA | NA | NA | NA |
It’s weird, it seems that there is a delay of ~ 2 hours of non-recorded data between each files…
Let’s load the smallest “already pre-processed” individual treated by
S. Cox (ind_140075
) to see what’s going on.
# load the data
ind_140075 = readRDS("../inst/extdata/cebc/Ind_140075/DSA_HauteRes/Pup_140075.rds")
# display the first rows
head(ind_140075) %>%
sable(caption = "First rows of the data already pre-processed of the individual `ind_140075`")
V1 | V2 | V3 | V4 | V5 | V6 | V7 | V8 | V9 | V10 | V11 | V12 | V13 | V14 | V15 | V16 | V17 |
---|---|---|---|---|---|---|---|---|---|---|---|---|---|---|---|---|
12/06/2014 18:28:11 | 0 | 0 | 0 | 0 | 0 | 197 | 0 | 45 | 255 | 5.9 | -5.75 | 0.8500004 | -8.55 | -5.45 | -2.50 | 0.0500004 |
12/06/2014 18:28:11.0625 | NA | NA | NA | NA | NA | NA | NA | NA | NA | NA | -5.45 | 0.9000004 | -8.65 | -4.85 | -2.35 | 0.0000004 |
12/06/2014 18:28:11.125 | NA | NA | NA | NA | NA | NA | NA | NA | NA | NA | -4.90 | 1.0000004 | -8.80 | -4.10 | -2.15 | -0.0499996 |
12/06/2014 18:28:11.1875 | NA | NA | NA | NA | NA | NA | NA | NA | NA | NA | -4.65 | 0.5500004 | -8.80 | -3.65 | -2.55 | 0.0000004 |
12/06/2014 18:28:11.25 | NA | NA | NA | NA | NA | NA | NA | NA | NA | NA | -4.05 | 0.5000004 | -9.05 | -2.90 | -2.55 | -0.1999996 |
12/06/2014 18:28:11.3125 | NA | NA | NA | NA | NA | NA | NA | NA | NA | NA | -3.45 | 0.5000004 | -9.30 | -2.15 | -2.50 | -0.4499996 |
…not the best import! Let’s convert the date and see if we can
identify the gap we’ve seen between 15/02/03 14:03
and
15/02/03 16:07
# let's (i) round datetime to second, (ii) select unique values, (iii) and sort
data_plot = data.table(datetime = ind_140075[, sort(as.POSIXct(unique(substr(V1, 1, 19)),
format = "%m/%d/%Y %T",
tz = "GMT"))])
# display difftime
data_plot[, diff_time := c(NA, diff(datetime))] %>%
ggplot(aes(x = datetime, y = diff_time)) +
geom_vline(xintercept = as.POSIXct("2015-03-02 16:07:00"), col = "red") +
geom_point() +
coord_cartesian(ylim = c(0, 10000)) +
labs(x = "Date", y = "Time difference between two rows (s)")
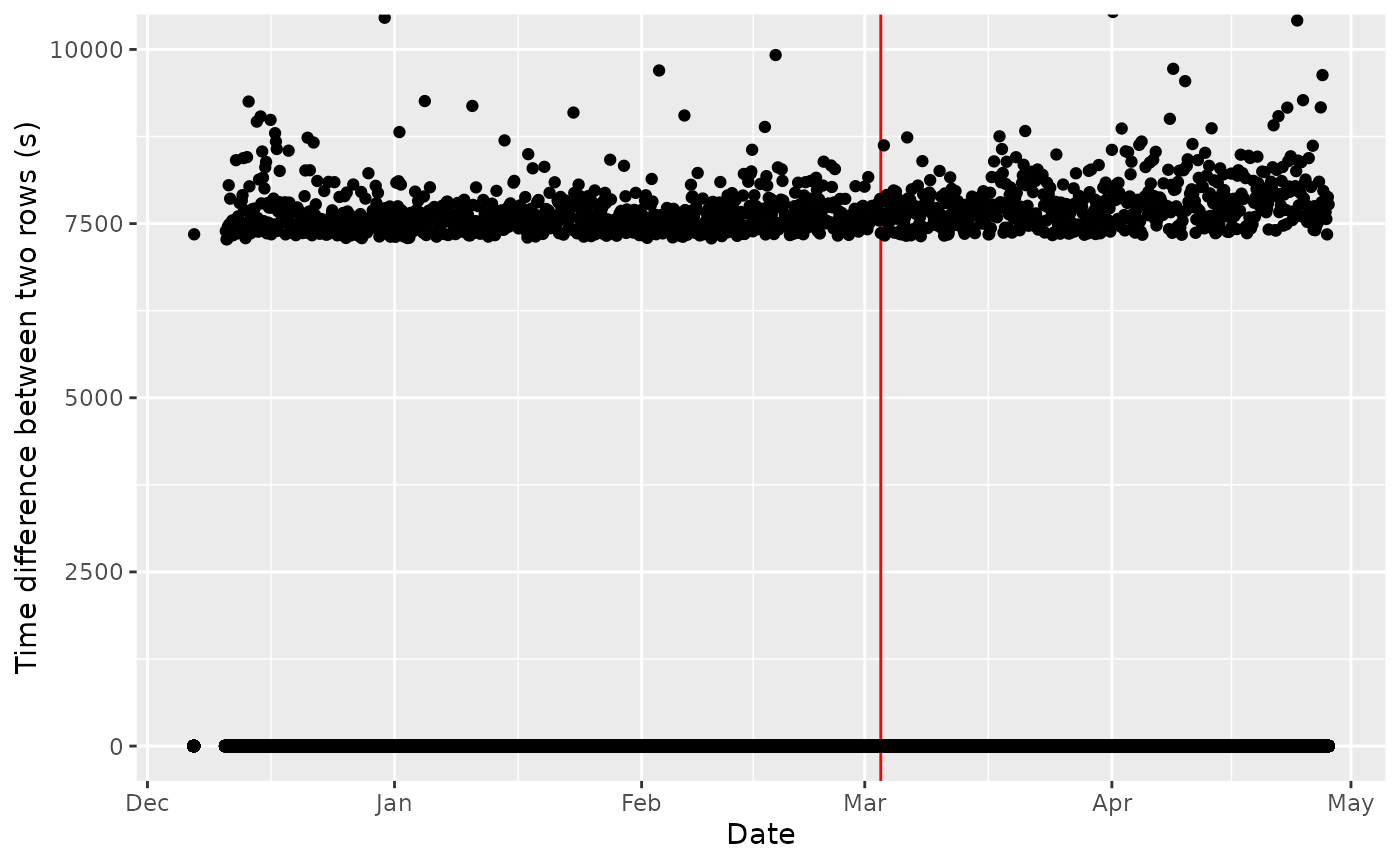
Time Gap Identification, if there were no time gap, all the dot should be at 1 second. The red line is the date when a new ’*.wch’ file has been created
Crap… We have 1459 gaps that exceed 1 s (in average of ~7959.553804s or ~
2.2
h). How do we deal with it? Is it the same for the other individuals?
# zoom over two days
data_plot[datetime %between% c(as.POSIXct("2015-03-01 16:07:00"), as.POSIXct("2015-03-03 16:07:00")),] %>%
ggplot(aes(x = datetime, y = diff_time)) +
geom_vline(xintercept = as.POSIXct("2015-03-02 16:07:00"), col = "red") +
geom_point() +
coord_cartesian(ylim = c(0, 10000)) +
labs(x = "Date", y = "Time difference between two rows (s)")
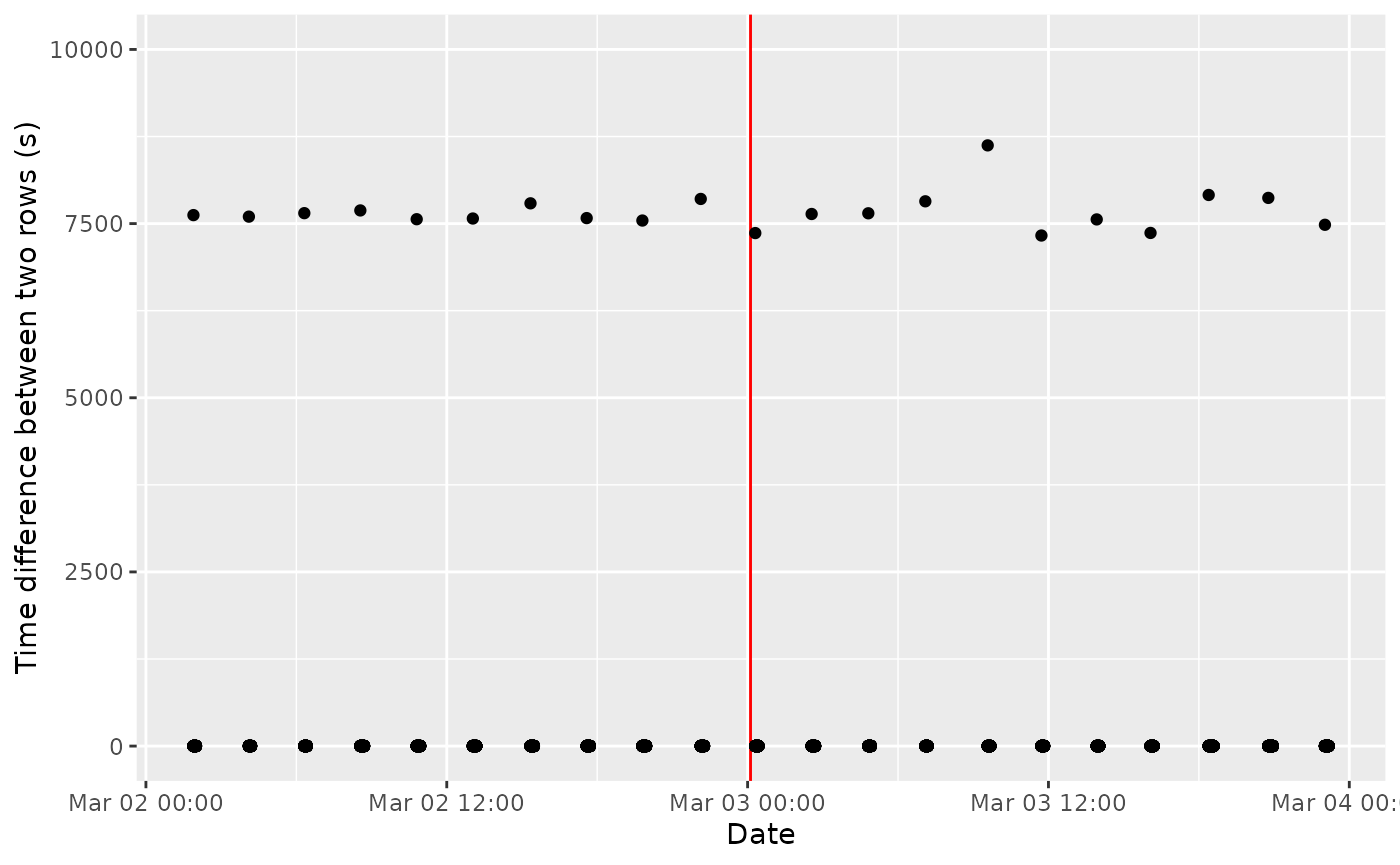
Same graph, with a zoom over two days
Well, there is clearly a pattern, I suspect the tag was programmed to be turned off every 2.2 hours?
Confirmed in Cox et al., 2017, the tag was programmed to record one complete dive every 2 hours!
# zoom over two days
ind_140075[!is.na(V2), datetime := as.POSIXct(V1, format = "%m/%d/%Y %T", tz = "GMT")] %>%
.[datetime %between% c(as.POSIXct("2015-03-02 12:00:00", tz = "GMT"), as.POSIXct("2015-03-02 18:00:00", tz = "GMT")),] %>%
ggplot(aes(x = datetime, y = -V6)) +
geom_point() +
labs(x = "Date", y = "Depth (m)")
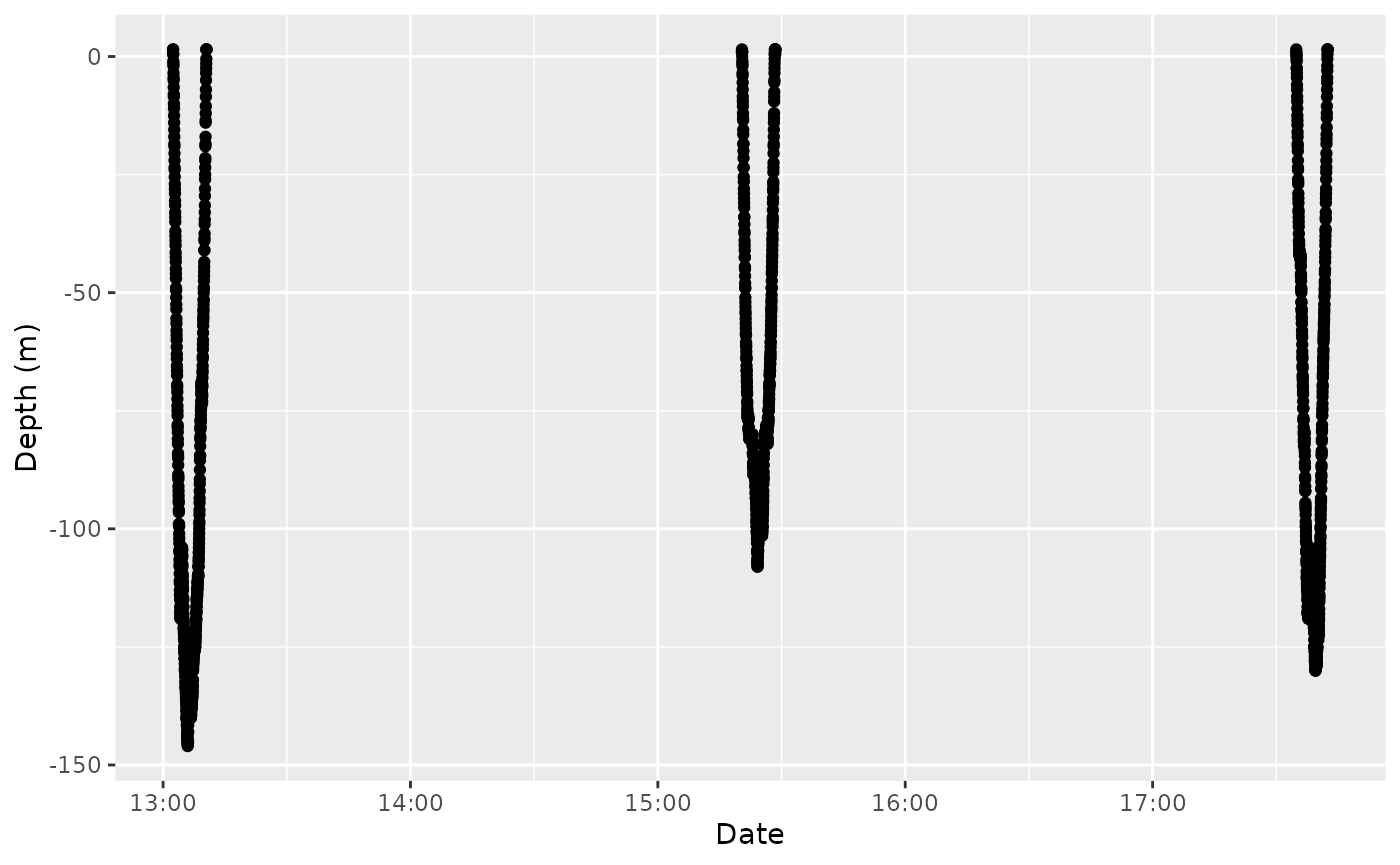
TDR over 6 hours of data recorded